Changing lives for good
as the world’s #1 health, wellbeing and navigation platform
-
1
Meaningful engagement
Drive daily interactions that create lasting behavior change, strong social connections, and improved wellbeing and loyalty
-
2
Better health outcomes
Close more gaps, manage chronic conditions and get your population the education and support they need
-
3
Easier
navigationDeliver a simplified experience that guides, connects and informs to reduce healthcare costs and optimize investments
6,000+ organizations, including 25% of the Global Fortune 500, in 190 countries and territories trust Virgin Pulse
-
For Employers
A full-stack health and wellbeing platform that strengthens your culture, motivates your people and boosts your bottom line.
-
For Health Plans
Data-driven engagement that educates and activates members of all ages and demographics, proactively closing care gaps and increasing satisfaction.
-
For Health Systems
Hyper-personalized campaigns that drive strategic growth for high-value services, boost referrals and support patient journeys year-round.
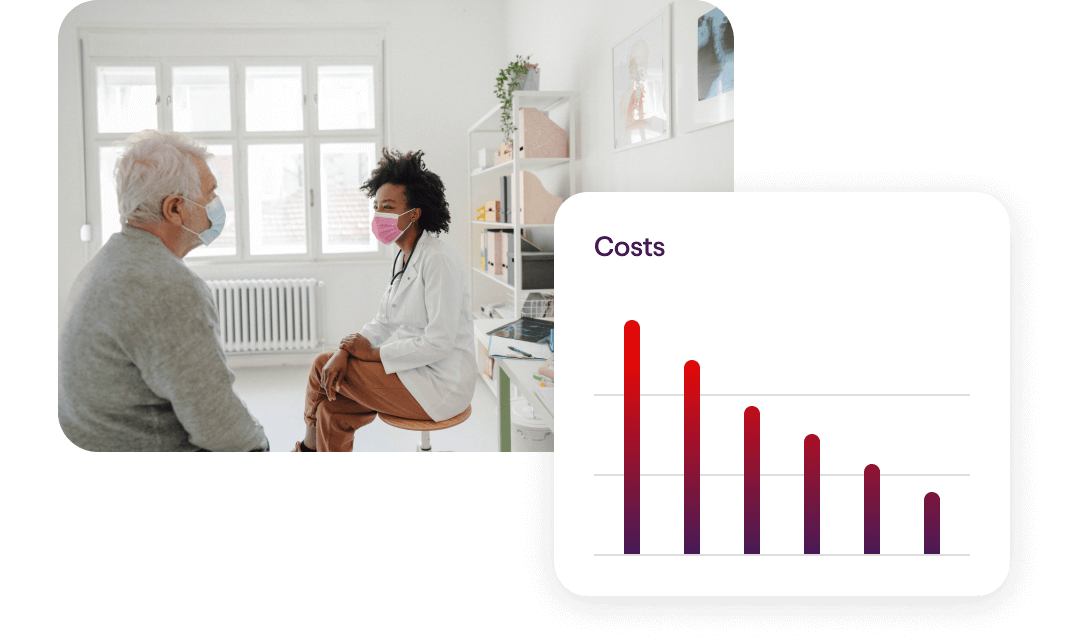
Reduce Healthcare Costs
Our unique combination of programs, partners and resources is proven to reduce healthcare costs by up to 27% annually for your population.
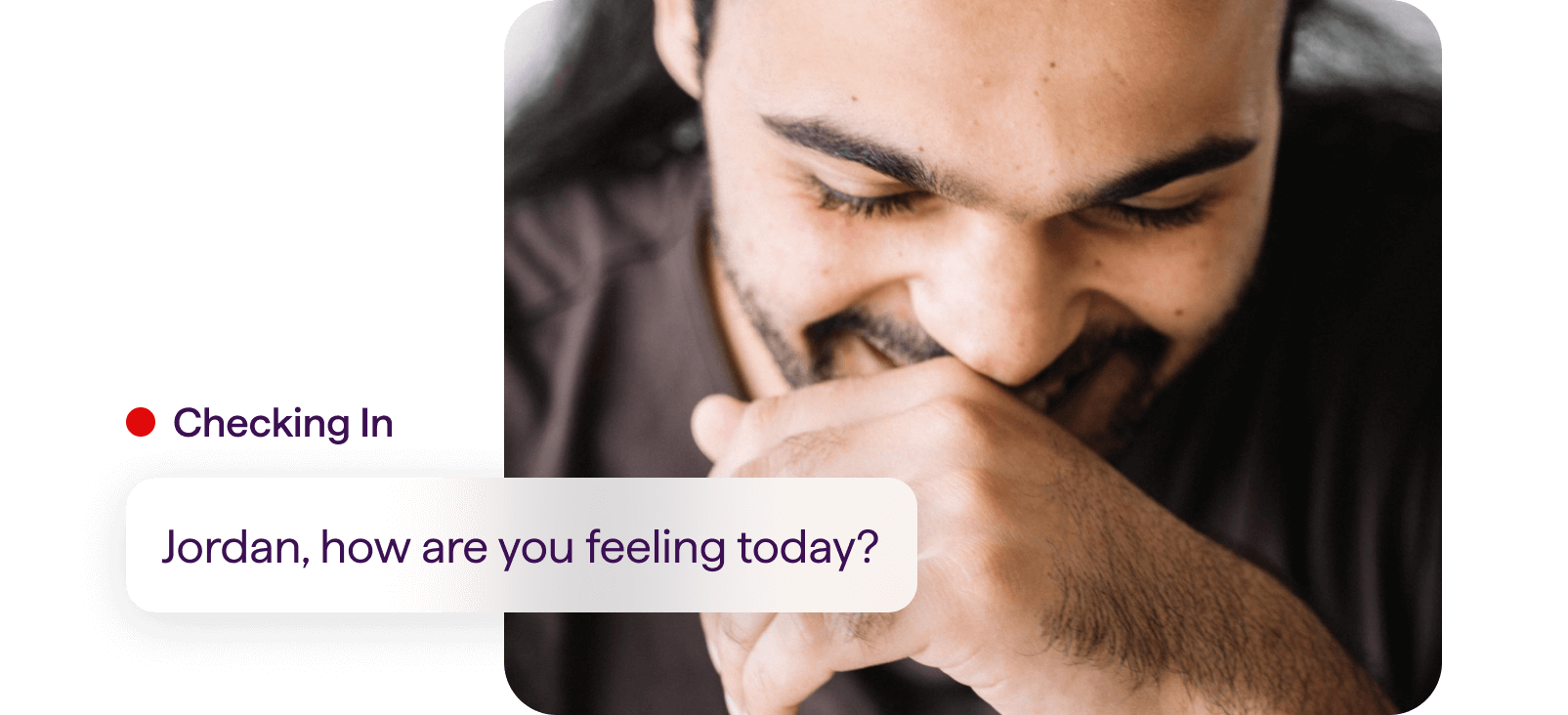
Boost Benefit Usage
Our AI-powered, personalization engine seamlessly connects people with relevant programs, benefits, tools and information through a centralized and simplified health and wellbeing platform they’ll love to use.
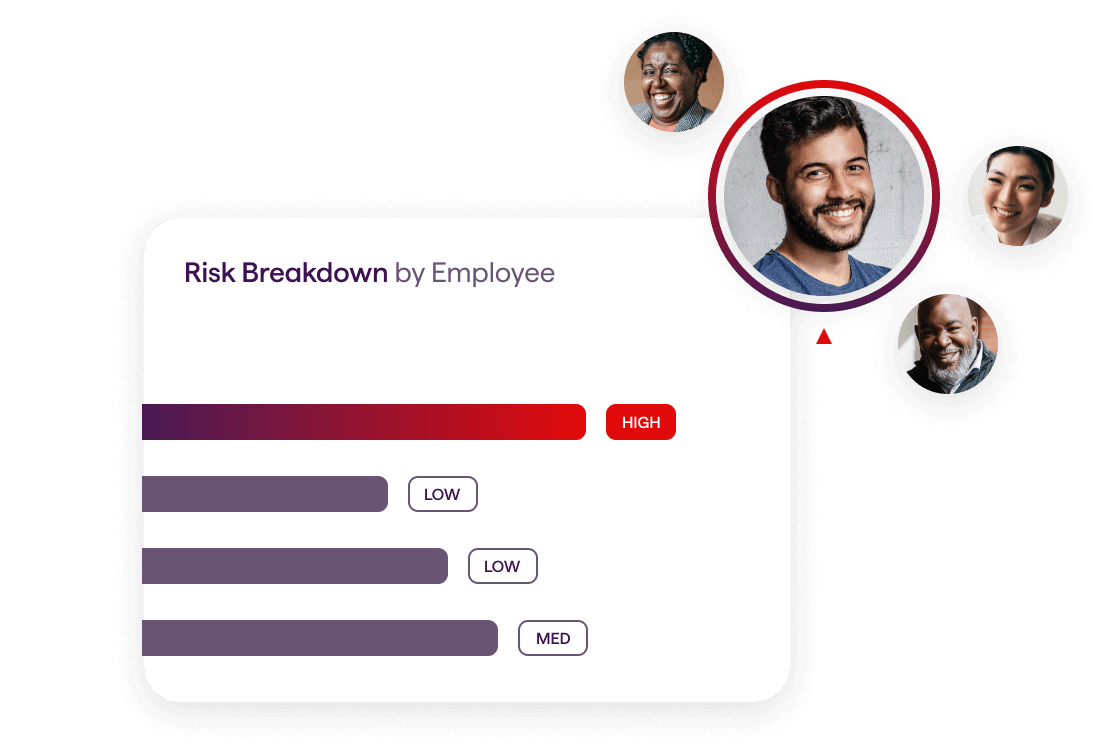
Improve Business Performance
Our end-to-end platform increases productivity and presenteeism by 44%, reduces safety incidents and attracts and retains top talent. Your people (and your bottom line) will be healthier and happier.
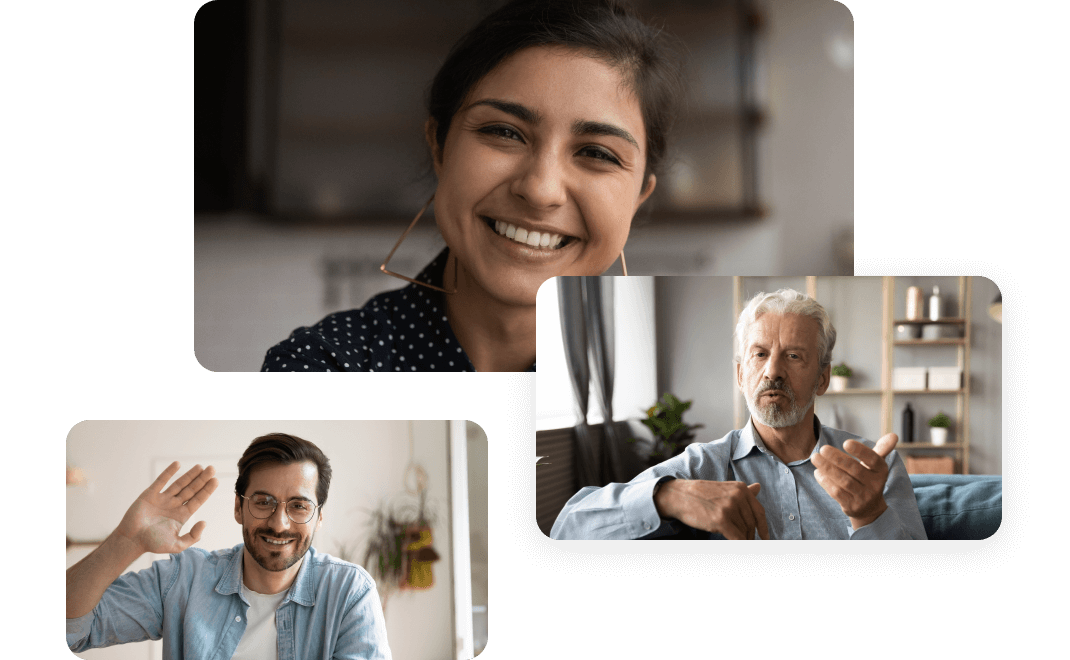
Foster an Empowering Culture
Our comprehensive digital front door is the gateway to building a great place to work, promoting your company values and DEI initiatives and strengthening connections across your organization.
-
87 say Virgin Pulse changed their lives
-
73 developed positive daily habits
-
68 improved clinical metrics
Latest Articles
BlogIt’s time to activate change.
Let’s Talk → → → →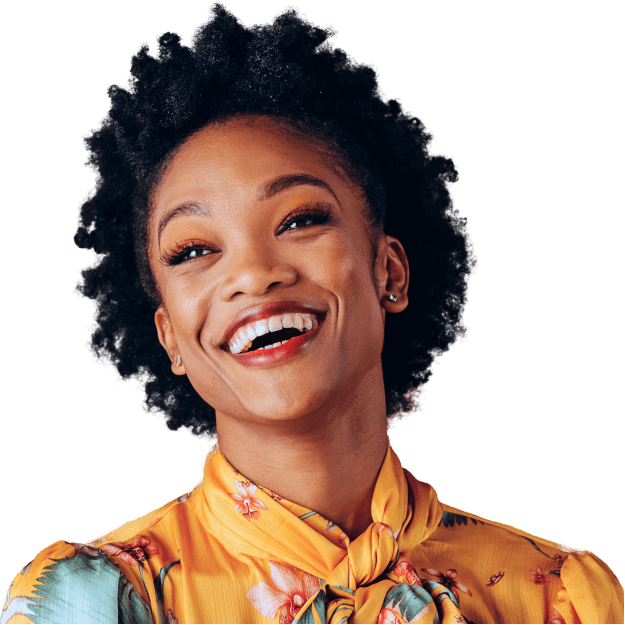